Ashish Raj, Ph.D.,
Associate Professor of Computer Science in Radiology
Associate Professor of Neuroscience, BMRI
Biography
I am currently Assistant Professor of Computer Science in Radiology and Co-Director of IDEAL, both at Weill Cornell Department of Radiology. I am an expert in algorithms, computer vision and graph theory applications in medical imaging. My research encompasses many computational problems in NEUROIMAGING, particularly the modeling of dementias using graph theory. We extract brain networks from neuroimaging data like Diffusion Tendor Imaging, functional MRI and MRI brain morphometry. The goal is to find network characteristics that distinguish healthy brains from pathological brains for a number of brain disorders like Alzheimer's, Epilepsy, Autism, Schizophrenia and Stroke.
I am also interested in the reconstruction of MR images from partially obtained data and on improving MRI resolution – parallel imaging and compressive sensing. I am the inventor of the EPIGRAM algorithm for reconstructing highly under-sampled data using graph-cuts.
I graduated with a PhD in 2005 from Cornell University in Electrical and Computer Engineering. I have more than 30journal papers ranging from microwave engineering, superconductivity, image/signal processing, vision, graph theory and neuroscience and hold three NIH grants (R01, R21, P41) as PI or Project PI. The defining characteristic of my work has been inter-disciplinarity: finding innovative ways to apply advanced theoretical methods to biomedical applications.
Click here for further information on Dr. Raj
Focus
I am now focused on cross-disciplinary innovation in life sciences in two broad areas:
A. Technique development in neuroimaging:
I was among the first to develop combinatorial algorithms like graph cuts for MR problems like reconstructing MR images from highly undersampled acquisitions. This gives up to 5 times speed up in brain scanning and is funded by NIH R21 and P41 grants. Since then my lab has applied this approach for brain image segmentation and myelin imaging via spatially constrained T2 relaxometry from multi-echo scans. We are also developing belief propagation-based global optimization algorithms for whole brain fiber tractography. The common thread in all these methods is the use of powerful spatial constraints for solving highly ill-posed problems in neuroimaging. Spatial constraints invoke prior expectations of what makes an image different from non-image data, and are able to produce impressive results from under-sampled or poor quality raw MR data. Our eventual goal is to create algorithms which can extract high quality information from neuroimaging scans in a clinically acceptable scan time. We will aim for extremely fast yet high quality diffusion MRI, tractography, T2 relaxometry, fMRI, perfusion MRI and structural MRI.
B. Brain connectivity networks in health and disease using graph theory:
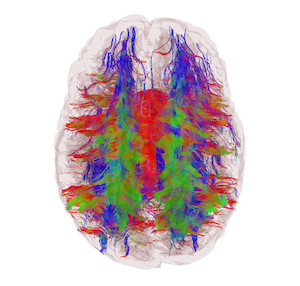
Our group was one of the first to apply brain-specific and disease-specific mathematical models involving brain connectivity networks. This is an active and crowded field with hundreds of papers showing small-world and scale-free networks in the brain, using summary statistics like clustering, degree and path length. Our lab is unique in not being interested in summary network statistics developed for social networks because they do not tell us anything specific about neural processes. We think that inordinate focus on social network-related approaches has distracted brain researchers from truly discovering important and fascinating features of brain networks.
We previously demonstrated largely uniform degree distribution in brain with surprisingly regular network organization (in contrast to previous reports of “hubs” in the brain). Next we showed that the total wiring cost is not only minimized by human brains but may also be responsible for the anatomic configuration of the cortical ribbon. We demonstrated a novel network approach to capture atrophy patterns in epilepsy, and to accurately classify disease groups. Then we used network disruption measures to relate white matter damage to gray matter disconnection, giving a highly informative metric for dementias.
Our recent Neuron paper mathematically models the macroscopic consequences of prion-like trans-synaptic spread of dementias in the brain in terms of an elegant network-diffusion model. The model implies the existence of a small number of distinct and spatially disjoint “eigen-modes” which correspond to the patterns of dementias like Alzheimer’s and frontotemporal dementia. This exciting work provides the first independent validation of proteopathy transmission and raises the intriguing possibility that various dementias are different modes of the same proteopathic mechanism. Clinically, this model can predict future dementia patterns of any patient based on baseline scans. This work was recently awarded a EUREKA R01 award from the NIH, and featured in some journal commentaries, including Nature Reviews Neuroscience, Nature Reviews Neurology, Neurology Today and Science Daily. Our next work will focus on prediction of future atrophy, which is one of the foremost goals of neuroimaging research in dementia.
Long term vision
I am committed to an agenda of exploring the full potential of mathematical models operating on brain connectivity networks. We hope to show that such models can explain almost all important observations about the brain, whether in health or in disease. For instance, we will use graph theory to elucidate how the resting functional connectivity networks are related to tractography-derived structural brain networks. The question about how the two networks are related is an important one in the field, but one that has bedeviled researchers for years. We believe that the success of our network diffusion model opens an exciting possibility for related linear network-based models to fill this gap. Ultimately, we expect that such models can capture how the brain functions as an ensemble of computational nodes connected in a complex but tractable network. In many ways, this is the primary goal of all computational neuroscience. In terms of disease modeling, we plan to employ graph diffusion and related models for Epilepsy, Parkinson’s, Autism and Schizophrenia. All these are network diseases, and should be amenable to the same approach that has worked in dementia modeling. The success of this ambitious but tractable programme will improve our understanding of the brain as a network processor, and how disease affects this network.